Business Intelligence Roadmap – Book Review
- Dr. Moria Levy
- Feb 1, 2009
- 21 min read
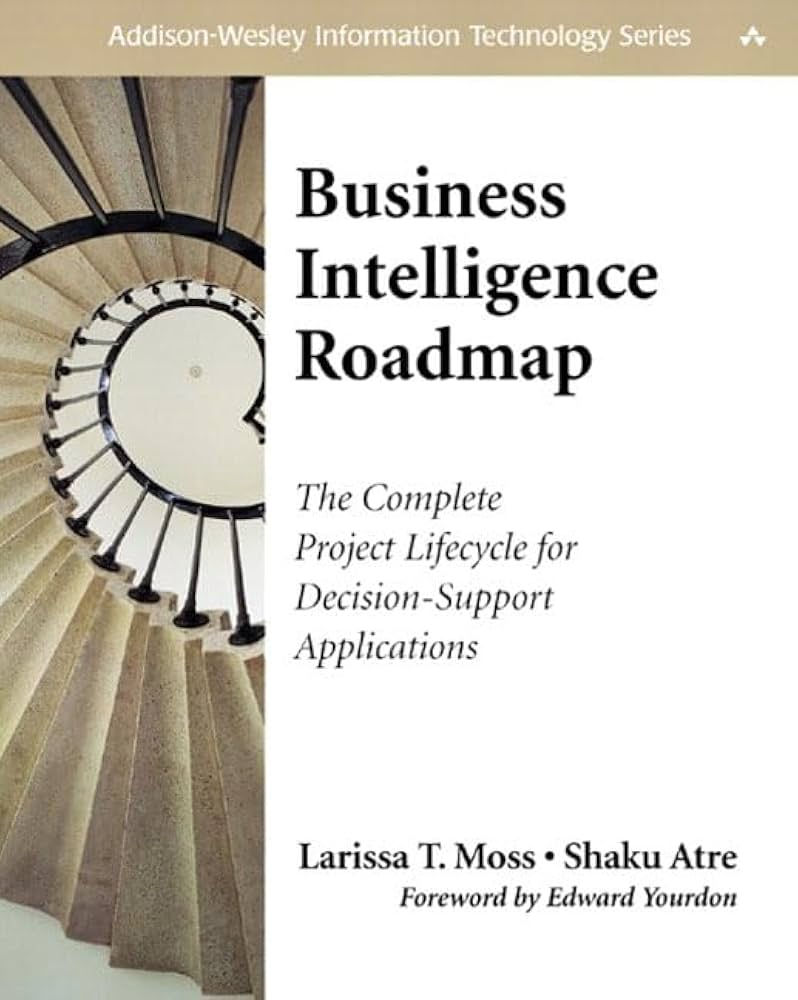
There is no doubt that the book "Business Intelligence Roadmap," written in 2003 by two women, Larissa T. Moss and Shaku Atre, is undoubtedly one of the most comprehensive books written in the field of business intelligence. The book deals with the business establishment of intelligence projects and reviews 920 activities that must be performed during this process.
The book has a technological orientation rather than a business one, and it's evident that information systems professionals wrote it, but certainly, experienced ones who have worked on dozens of projects at least, if not more. In addition to the 16 chapters describing each of the different stages of activity, additional chapters review the activity from various perspectives: who are the partners and in which stages do they take part; a list of tips and do's and don'ts for each stage; entry and exit points for each stage, teaching, beyond the results obtained from performing the stage, when it's not advisable to perform the stage (when the entry point is not met, or when the exit point has already been achieved in the organization); a detailed work plan, and more.
The sixteen stages are organized into six super-stages for establishing the business intelligence environment. It doesn't seem that many will adhere to this level of input and detail. Nevertheless, there is something to learn from understanding the proposed model:
Main topics covered:
Justification
Business Case Assessment
Planning
Enterprise Infrastructure Evaluation
Project Planning
Business Analytics
Project Requirements Definition
Data Analysis
Application Prototyping
Meta Data Repository Analysis
Design
Database Design
ETL Design
Meta Data Repository Design
Development
ETL Development
Application Development
Data Mining
Meta Data Repository Development
Layout
Implementation
Release Evaluation
I chose to briefly detail advanced information and tips for executing the various stages. The basic information (what the stage is) and the in-depth information (many details on how to manage it) should be read in the book itself. As one might expect, the summary includes only a fraction of the content (the whole book is 543 pages), and anyone involved in new or experienced business intelligence will learn from it. Despite lacking essential chapters (implementation, change management, connection to work processes), this is. Things that seemed to me as a reader to be worthy and less trivial were marked with a special symbol. The bottom line is that this is a comprehensive and highly recommended book.
I hope you have an enjoyable read.
Justification of the Activity
Business Case Assessment
Establishing an accurate and comprehensive business intelligence environment costs millions of dollars. No less.
Such an environment, in part or in full, should not be established without business justification, initiative, desire, and understanding of the business needs of the company's business people. The justification for business intelligence activity must always be business-driven, not technology-driven, and it's usually an iterative rather than a one-time activity.
Here are several categories of expected business benefits:
Increasing revenue.
Increasing profit.
Improving customer satisfaction.
Reducing costs.
Increasing market share.
For each of these, focused business benefits that can be achieved through business intelligence are detailed.
But there's no benefit without cost, and in the case of business intelligence activities, each activity is also accompanied by risks, more than in other IT activities.
Types of risks to prepare for:
Technological risks: Maturity of technologies in the market and the organization; number of technologies required; incompatibility of operating systems; incompatibility of databases.
Complexity risks include the complexity of the IT environment, the complexity of the business intelligence solution, the expected frequency of process changes, the expected number of sites, and the level of decentralization of data, processes, and control.
Integration risks: How many interfaces are expected to be implemented; are there external interfaces; how much data duplication is expected; how many primary keys need to be integrated into the implementation; are there conflicting standards; are there standards at all; are many orphaned records expected, contradicting data integrity rules.
Organizational risks: How risk-tolerant is the organization, how risk-tolerant is the IT management, and how much moral and budgetary support can be expected if the project encounters difficulties?
Team risks: How much experience do team members have in implementing BI projects; how well-founded is the experience; how balanced is the team; what is the level of team cohesion and morale; what is the risk of a team member leaving; do the team members have all the required skills and knowledge; how active and initiative-taking will the business representative be; how strong is the project manager.
Financial risks: To what extent can ROI be expected; what is the risk that costs will outweigh benefits; can expected risks be overcome due to technological improvement?
This stage includes the following business activities: defining the business need, understanding the current situation, setting goals, proposing a business intelligence solution, cost-benefit analysis, risk analysis, and writing a summary report.
Tip: The project and initiative are most likely to succeed if they are led by the business entity and its representatives.
Planning
Enterprise Infrastructure Evaluation
Business intelligence activities focus on establishing an organizational infrastructure that supports decision-making. The lack of a cross-unit organizational perspective creates a "spaghetti" effect and, in the long run, reduces the chances of managing the organization based on its data. Understanding the organization's infrastructure in advance is important even before starting solution development to understand what complex yet information-sharing environment we are entering and what the chances of success for cross-organizational activity in the long term are as a result of these factors.
The infrastructure analysis includes two parts:
Technological Analysis:
Examines:
Hardware, its distribution, and the level of control over this distribution and data allocation within it.
Middleware and the current information and data transfer between different systems and units.
Database Management System (DBMS), its level of diversity (for different types of databases), and the technological level of the leading databases.
Includes the following activities:
Evaluation of the existing platform; evaluation and selection of additional infrastructure products; infrastructure report; acquisition and expansion.
Organizational Analysis:
Examines the current state of:
Functional organizational activities.
Work processes.
Entities and data.
Use of operational systems for information. Cross-organizational information.
Infrastructure data dictionary.
Working according to standards and uniform standards.
Includes the following activities:
Evaluation of organizational infrastructure; status report; improvement.
A significant statement: If the organization has patience and tolerance, it is advisable to start by improving the computational and organizational infrastructures and only then begin direct business intelligence activities. Of course, the benefits are clear, but not every organization can start in this spirit. In such cases, it is recommended to incorporate at least one activity in each direct BI activity to promote the organization’s goal of adding standards/infrastructure unification.
Tip: If there is an organizational data dictionary, it's advisable to use it for analysis. If not, and there is a CASE tool, it can provide much of the data needed for evaluation.
Project Planning
Planning a business intelligence project is different from planning a regular project. The reasons:
Different skills and experience are required than those involved in managing an operational system information project.
Extensive hands-on capabilities are required to manage the activity.
During the project, more changes than usual are required. Schedule postponements are frequent, and one should get used to them and prepare accordingly in advance.
Extensive iteration and repetition of similar stages with different content are required as an integral part of the activity.
It is recommended to define (and write a report) that includes reference to the following aspects:
Goals and objectives
Scope
Risks
Constraints
Basic assumptions
Change management processes
Management issue handling processes
It's worth noting that this is only part of the regular management components of any activity, a part that receives extra weight in business intelligence activities.
Points to consider:
Note that the project is data-intensive and not necessarily functionality-intensive. Prepare accordingly in scoping.
Common risks: lack of commitment, lost sponsor, lack of cooperation from the business side, unrealistic schedule, unrealistic scope, excessive expectations, inadequate budget, an unskilled team lacking sufficient training and knowledge, frequent changes in business prioritization, inefficient project management, limited upgrade, and expansion capabilities.
Constraints: There are five typical constraints in any activity: quality, budget, resources, time, and scope. It is recommended to ensure that this is also the order of importance in addressing them (quality first, scope last, and the rest in this order).
Project planning includes defining requirements, defining the state of databases and source files, re-examining cost and risk assessment, defining critical success factors, preparing a project plan, preparing a master work plan, and Kickoff.
Business Analysis
Project Requirements Definition
The requirements definition activity takes on a different hue when dealing with the overall business intelligence activity in the organization and its initiation, as opposed to focusing on a specific business intelligence activity for a defined target audience and purpose.
In the first case of initiating comprehensive activity, it is recommended to prepare a report including the following:
Requirements by topic (each will likely develop into a project later);
A list of critical business issues that arose during the interviews and propose to address them immediately, whether through business intelligence activities or other activities (often other needs arise along the way);
Opportunities that can be identified and should be leveraged in business intelligence activities;
Recommendations.
Next steps for action (indicating what is more critical).
In the second case of focused business intelligence activity, it is recommended to prepare a report including:
Objectives in terms of:
Nature of the business problem.
The damage caused by the absence of a supporting BI environment (cost of not managing information).
Why the problem cannot be solved without business intelligence.
How business intelligence helps solve the problem.
Detailed requirements of:
Data; information sources.
Required tools.
Reports and graphs; functionality.
Data cleansing (prioritizing what is more critical).
Historical information.
Information security.
Proposed Service Level Agreement (response times, availability, cleansing level, etc.) SLA.
The business activities included in this stage: Defining technical infrastructure requirements; defining non-technical infrastructure requirements (standards, etc.); defining the content of the requirements document; defining requirements from information sources; reviewing the scope of activity; expanding the logical data model; defining SLA; writing a detailed requirements report.
Tips for interviews on which the above reports are based:
In initial interviews, it's recommended to avoid going into details. Focus on the business aspect.
People tend to talk about what they have more than what they need.
There will be conflicting opinions.
Avoid writing too much during the interview. It disrupts the flow of conversation.
It's worthwhile to pass the interview summaries to people and ensure that the needs were understood as we thought.
Additional tips: Ensure that the scope is feasible; separate the definition of requirements from the design by software people; avoid excess data just because "we have it."
Tips:
Changes during the project will happen, whether we want them or not. Choose a project manager who will know how to deal with them. Be careful, as unwise change management can kill the entire project.
Many activities can be performed in parallel. It is recommended that you take advantage of this, as time will always be in short supply.
Data Analysis
The book authors define this data analysis stage as the most critical in the entire activity.
It is recommended to conduct data analysis by combining both well-known analysis methods:
Top-down analysis from above is the best way to understand the overall picture of the organization.
Bottom-up - analysis from the perspective of each project separately while integrating the data into the existing data array.
Emphasis should be placed on ensuring that the data analysis is independent and not dependent on the database, hardware, access method, design, tools, or programs.
The authors express a wish that can save us a lot of resources in setting up business intelligence systems: defining a mandatory standard, according to which any operational system development requires the development of a logical data model in parallel. This will be integrated into the business environment's data model and save a lot of money, time, and suboptimal thinking compared to the current situation.
A good data model includes for each data point:
Name, description, link to business activities, key, type, length, content, business rules, governance policy, ownership.
When we talk about a complete model, we define:
Technical conversion rules between systems. Always apply.
Business rules related to the data content. Details below.
Data integrity rules. Details below.
Violations of business rules:
Missing data values.
Use of default values (0, 999, FF, etc.).
Dummy values with unique business meaning (using ID 888 to indicate a foreign resident, etc.).
Logic included within data values: low postal codes indicate the settlement area in the country.
Multiple uses of one field: values in a particular range refer to customer type, values in another range refer to location, etc.
Multiple uses over time of the same data for different purposes (redefine in COBOL language).
Data interpreted over more than one field (address in 5 lines constituting five fields).
Violations of data integrity rules:
Contradictions between data related to a shared entity. For example, London, France.
Violations of defined business rules for the same entity. For example, a person whose date of birth is after their date of death.
Different values with a uniform key (two people with the same ID).
Lack of a unique key (one customer with several different customer codes).
Entities without a parent entity. For example - an employee with a non-existent manager code.
Data Cleansing
One of the goals of a business intelligence environment is to provide integrative information that resolves contradictions between different sources. This cannot occur if the data is not cleansed. When selecting data for the business environment, this should be considered. Selection stages:
Identifying required data, analyzing data content, selecting appropriate data (only the most core that meets the needs), verifying a reasonable level of cleanliness, preparing a cleansing specification, and selecting supporting tools.
Key points when selecting data and understanding their cleanliness level:
Level of data completeness. The more data is manually entered, the greater its completeness.
Level of data accuracy (significantly incomplete numerical data).
Level of data correctness (whether the checks on data entry and maintaining their correctness are known).
Level of data reliability. The older the data, the more likely it is no longer reliable, whether it's source data or a copy of data.
Basic data structure. It will be easier to convert and ensure cleanliness on a tabular source than a hierarchical one.
The business activities included in this stage are analyzing external information sources, improving and expanding the logical data model, analyzing the level of data cleanliness, resolving contradictions, and defining cleansing specifications.
Tips:
Trivial, but not always performed; involving a DBA in performing this stage.
Within the project scope, the Top-Down technique will be used to define business rules, and the Bottom-Up technique will be used to detect violations.
For managers who haven't managed such a stage, multiply all time estimates by 4!!!!
Application Prototyping
The prototype stage is adequate for understanding gaps and resolving hidden contradictions.
Lessons learned from creating prototypes:
Limit the scope.
Understand database requirements as early as possible. It will affect database design.
Choose the correct data to incorporate into the prototype. Choose a small enough sample.
The user-friendliness and convenience of access and data analysis tools will be examined at this stage.
Involve business stakeholders in the process.
Recommended points when determining a prototype:
Small prototype team.
Tight deadline management.
Scope. Use the term - slimware.
Well-defined deliverables.
Content: This includes testing of GUI, interfaces, and additional output methods.
Minimize data integrity definitions for the prototype.
Involve up to 5 (and in exceptional cases, up to 8) business stakeholders in the prototype—no more.
Encourage business stakeholders to define success metrics.
Known types of prototypes
Show & Tell (management-oriented); Mock-up (for understanding data access and analysis methods); Proof-of-Concept (for clarifying unresolved implementation points); Visual Design (like Mock-up, but more in-depth); Demo (shows partial functionality); Operational (examines operational issues). Broad).
It is recommended that the prototype be accompanied by a defining document that includes the definition of type, objectives, involved business stakeholders, hardware and software, and requirements to ensure standards, team knowledge and skills, and usability.
The business activities included in this stage are access requirements analysis, scope definition, selection of prototyping tools, preparation of the defining document, definition of reports and queries, building the prototype, and presenting the prototype.
Tips:
Demonstrate the prototype several times in the process to partners and opinion holders.
Business stakeholders will be the ones to examine user-friendliness and convenience.
Many managers think they only want summary results. Usually, it turns out differently. Examine this point in the prototype.
Meta Data Repository Analysis
A metadata repository is also a database, but its purpose is to contain information about existing data. The information serves as a tool for understanding (comprehensive of the array and specific of the data state), as a management tool, and as a navigation tool in content. The existence of a data dictionary helps promote standardization.
The recommended information included is classified into four groups:
Ownership (of data and applications).
Descriptive characteristics (for business processes; for business data): name, description, definition, allowed values, notes.
Business rules and policies: relationships, business policy, information security, cleansing, validity, currency.
Physical characteristics (for data and applications): source, physical location, conversions, various calculations, scope, and growth.
There are many challenges related to the data dictionary:
Technical challenges. It's a project. Decide whether to buy or build in-house.
Staffing challenges. An administrator must maintain the infrastructure dictionary; otherwise, it will get disconnected.
Budgetary challenges. Organizations don't want to invest (or invest enough) in setting up and maintaining the dictionary.
Usability challenges. Most infrastructure data dictionaries are not convenient enough for use and accessibility.
Political challenges. Departments' handling of business intelligence varies Greatly. Uniformity is mandatory here.
The business activities included in this stage are analyzing infrastructure dictionary requirements, analyzing interface requirements, analyzing access and reporting requirements from the dictionary, building a logical data model (ERD diagram), and defining the type of data to be managed.
Tip: It can be challenging to set up an infrastructure dictionary simultaneously. Prioritize the type of information to be stored: mandatory, necessary, possible.
Design
Database Design
Databases supporting business intelligence activities differ from those supporting operational systems.
Main differences:
Oriented towards different users with different needs. Redundancies are allowed.
Response times are measured in seconds, minutes, and hours (not sub-seconds).
Not normalized!
Contains much-calculated information, not just raw data.
Contains historical information, not just current snapshots.
Include many levels of summary information.
The main types of database design include:
Star Schema for tabular storage of cubic data.
Snowflake Schema - same as above, but creating a hierarchy for access attributes (e.g., country > city > neighborhood).
Physical design is essential to ensure reasonable response times. Options for speeding up response times:
Storing frequently accessed data on fast devices.
Storing different levels of summaries on different platforms.
Disk stripping (to physically smaller disks) to achieve optimization of input-output activity.
Positioning datasets to avoid lengthy searches as much as possible.
Selecting address and retrieval schemes that shorten the number of seeks. The goal - one seek for each retrieval.
Running as many activities as possible in parallel.
Separating indexes from data. Separating the indexes themselves between different disks.
Physical design includes consideration of the following parameters:
Partitioning, Clustering, Indexing, Reorganizations, Backup & Recovery.
The business activities included in this stage are reviewing data requirements, deciding on required summary levels, designing the logical database, designing the physical database structure, building the databases, developing maintenance processes, preparing for monitoring and improving design, and preparing for monitoring and improving query design.
Tips:
Let the DBAs design the database. Don't decide alone and leave them only with the building.
Instill the understanding that business intelligence databases are massive (VLDB). Those that aren't - will be. Plan accordingly.
Clustering improves performance significantly. Take advantage of it.
Don't build indexes when values refer to more than 15% of the data (gender).
Reorganize the database after 5% additions/deletions.
ETL Design
ETL, or Extract-Transform-Load in full, deals with the correct retrieval of data from various operational systems. The core of this stage is, in its middle part, the transformation, which is also responsible for data cleansing. The most crucial rule in implementing ETL is the existence of one such collaborative process (and not distributed activities).
Preparation for the ETL process includes:
Reformatting - reformatting, as information sources are of various types.
Reconciling - resolving contradictions between the multiple sources combined.
Cleansing - preparing for data cleansing: defining the needs.
ETL includes three types of programs: initial loading, historical data loading, and gap loading. The design should address all these types.
Extraction: From the operational systems' perspective, it's simplest to duplicate the data and let the business intelligence array handle the rest. From a business intelligence perspective, the preferred way is sorting, filtering, cleansing, and summarizing directly at the source as one action, resulting in the extraction. A compromise is usually made: the extraction is a duplication, but one that allows reaching the desired information relatively in the fastest way possible.
Transformation is the most complex part. It's necessary to address problems in the source data—inconsistent key data, inconsistent values, different data fonts, inaccurate data values, synonyms and multiple names for a single term, and logic hidden in code.
Loading: Loading of clean data. Canceling RI relationships and indexes before loading and restoring them afterward is recommended.
This stage includes the business activities of writing a source-target mapping report, examining ETL tool capabilities, designing the ETL process, designing ETL programs, and preparing a workspace for ETL.
Tips:
ETL is the most complex process in all activities of setting up a business intelligence environment, especially the transformation. Be sure to allocate enough time for this stage.
The biggest challenge in designing good ETL is finding people who understand the meaning of the data, business rules, and history. This research should involve both business stakeholders and relevant programmers from operational environments.
Meta Data Repository Design
Designing a data dictionary is not a new issue; it has been common since the early 1980s. However, it has always been characterized by many problems, from manual input through immature technologies to failure to create a shared, cross-unit dictionary with organizational understanding and appreciation.
This is not to say that the situation has reversed. Still, there is improvement, at least in some dimensions, and considering an infrastructure data dictionary is certainly possible.
Key issues in designing a data dictionary:
Centralized / Distributed (shared model, distributed information) / XML-based (different models in different dictionaries, with shared XML tagging) dictionary. Considerations for decision-interface convenience, content control, reliability and automatic updating, ownership, and data redundancy.
Purchase / In-house development: Considerations for decision-making that fit needs and save development resources.
ERD (Entity Relational Diagram) / OO (Object-Oriented) structure: Considerations for decision: ease of reading and understanding, structural flexibility, simplicity of retrieval, and simplicity of implementation.
The business activities included in this stage are designing the database of the infrastructure data dictionary, installing accompanying products (or development), creating the process of transferring data to the dictionary and designing required application programs.
Tips:
Start with a central data dictionary because it's easier to set up and maintain.
Most approaches to the data dictionary should be by business stakeholders and not by technical people. Plan accordingly.
Development
ETL Development
Many of the previous stages involved collecting business rules. These are implemented in this stage of building the actual conversion.
The conversion includes the following components:
Data cleansing.
Summaries (mainly sums or quantities).
Calculated data.
Aggregation of data from various sources.
Data integration and decision on keys and unique names.
One of the claims heard from business stakeholders against business intelligence environments is a lack of data reliability. Ironically, when there are discrepancies, the data in business intelligence environments is usually more accurate, not less. However, this requires proof, and keeping information about the conversion and transfer time is critical for this purpose. Proper data transfer is also recommended to be verified (and this information stored in a place accessible to stakeholders) to prevent real reliability issues.
Business activities included in this stage are building and unit testing of the ETL process; integration or regression testing of the process; performance testing of the process (!!!); quality testing; and acceptance testing.
Tips:
Organizations invest about 80% of their business intelligence activity time in "backend" efforts, including data cleansing. Tools can help assess and improve cleanliness.
80% of the investment in data cleansing is in business rules and only 20% in technical conversions. Choose where to invest efforts in the first part to create a 20-80 split (20% effort that will address 80% of the need).
The most common symptoms of unclean data are data contradictions and reusing the same field for different purposes. The second component is ubiquitous in flat file extractions.
Application Development
Application development includes setting up an environment with ready-made and easily accessible templates. This includes calculations and summaries, but no less importantly, prepared cubes that are easy to navigate to reach the desired information. It is recommended that ready-made OLAP tools be used in this environment.
The functionality associated with OLAP tools:
Represent information and access to it in a multidimensional manner.
Provide summary and aggregation.
Provide interactive query and analysis capabilities.
Support business analysts, designing variable queries.
Support drill-down, roll-up, and drill-across operations.
Include analytical models.
Include trend analysis models.
Present information in reports and graphs.
OLAP infrastructure includes display services, OLAP services, and database services.
Many organizations deal with customer-related business intelligence. The eight dimensions related to customers and information about them are:
Customer type
Purchase behavior
Credit rating
Domain
Demographics
Psychographics (market segmentation based on personality traits of target audiences)
Purchase history
Product category
This stage includes the following business activities: final decision on requirements; design of application programs; building and unit testing of programs; comprehensive testing of programs; and training for information access.
Tips:
Adapt the display to the stakeholders' literacy and habits. Don't provide an overly sophisticated solution to those not suited for it.
It's easy to understand 2-3 dimensions; 5-6 dimensions are difficult to grasp; 7 dimensions are the maximum.
To enable reasonable performance, prepare summary information for common queries. This is more effective than explaining why response times are lengthening.
Data Mining
Data mining is not off-the-shelf software. It requires, alongside software tools, a supporting application. Data mining is not statistical analysis and differs from it in several aspects:
It does not require a preliminary assumption (which is then tested).
The algorithms develop the equations to be tested on their own.
It can work on non-numerical data as well.
It requires clean, well-prepared data to function correctly.
The importance of implementing a data mining solution lies in its ability to answer questions that decision-makers don't know how to ask. Therefore, it is also commonly referred to as Knowledge Discovery and is a complementary component to the previously mentioned capabilities, not a replacement.
Often, despite a data warehouse serving as an environment for the business intelligence system, it's worth considering working with the operational information system if the business system includes summaries rather than raw data (which makes its operation difficult). On the other hand, if the data in the operational systems is not clean, using the business intelligence environment might be preferable.
Data mining techniques
Associations Discovery - Identifying behavior of discrete events/processes (supermarket purchases).
Sequential Pattern Discovery - Identifying behavior over time of an event/process (supermarket purchases).
Classification - Identifying characteristics of predefined groups (characteristics of frequent flyers).
Clustering*- Organizing items into groups; identifying the group to which each item belongs.
Forecasting - Regression analysis in general and time-dependent regression analysis in particular.
The main applications of data mining are market management, fraud prediction, risk management, financial services, and distribution (inventory control).
This stage includes the following business activities: defining the business problem, data collection, data merging and cleansing, data preparation, building the analytical model, analyzing results, performing external validation tests, and monitoring the model over time.
Tips:
Compare the results you obtained to benchmarks or other external information.
Any data mining activity that has no use for its results does not support cost-effectiveness. Think ahead.
Meta Data Repository Development
An infrastructural data dictionary serves technical people, but much more so, it serves stakeholders. If you don't acquire one, you need to build it yourself. Beyond that, you need to plan the collection of information into it from various sources:
Documents - procedures, manuals, and additional documents describing business rules.
Spreadsheets (Excel) - for calculations, macros, and more information.
CASE tools - including definitions of data stored in databases.
DBMS dictionaries - as above.
ETL tools - including information about transfers.
OLAP tools - including information about calculations and summaries.
Data mining tools - including descriptive information about the analytical models in use.
When purchasing or developing an infrastructural data dictionary, ensure it includes a user-friendly interface for access and navigation for all stakeholders, from managers, other decision-makers, and experts performing analyses to technical people.
Business activities included in this stage: Building the database for the dictionary; building and testing the process of transferring information to the dictionary; building and testing the dictionary application; testing the comprehensive programs or the ready-made product (with data); preparing the dictionary for production; training.
Tips:
Building an infrastructural data dictionary is not a one-time event but an evolving process.
When developing a dictionary on your own, prepare to build two types of interfaces: one for programs loading the dictionary and one for stakeholders using it.
The size of a data dictionary can be up to 5% of the database size if built correctly. Check yourself.
For every day of building, allocate three days for testing.
Layout
Implementation
After planning, building, and testing the business intelligence system, we reach the anticipated stage of making it available to users in the production environment. It is recommended to perform a gradual launch and expose the environment to expanding circles of users each time after examining, approving, and making appropriate environmental adjustments.
Permission management and data security: Permission management and maintaining data security are manual tasks that cannot be avoided in the business intelligence environment; no ready-made umbrella preserves the operational system settings and restores them in the new environment.
Backups: Prepare for regular backups of the business intelligence system. This can be done using one of three methods:
Incremental backup
Rapid backup of operational system data from which information is retrieved
Partial backup of different data areas each time (while the rest remain available when not being backed up)
Monitoring: Continuously monitor the computer systems, networks, and response times of executed queries.
Growth: As a rule of thumb, a business intelligence system doubles in size every two years. Prepare for growth in data, usage, and hardware. This stage includes business activities such as implementation planning, setting up the production environment, installing all application components, uploading ETL programs to the regularly executed program schedule, loading production data, and preparing for support.
Tips:
Data proportions: 30% business data, 30% indexes, 30% summaries, and 10% other.
Release Evaluation
The version concept divides each business intelligence project into information brought now and information to be brought in the future. This approach allows for solution deployment; otherwise, we too often postpone the launch and invest 80:20 in things that may not always be necessary and certainly not needed now.
Principles for defining a version:
It is recommended that a new version be released every 3-6 months (except for the first one, which takes longer).
Version products should be small and manageable.
Manage expectations regarding what is received in the current version and what is not.
A version does not have to include a complete implementation.
For the first version, preferably provide only foundations.
Management should be prepared to receive information in versions.
Everything is negotiable. Nothing is "mandatory."
The supporting infrastructure should be stable.
Metadata is part of every version; otherwise, it cannot be managed.
The development process should be visible.
Tools should support the flexibility required for working in versions (gradual development).
Prioritize new needs and not necessarily fulfill them in the order they arrive.
Small errors should be fixed between versions without delay until the next version; large errors will wait, and if necessary, the incorrect information/functionality should be removed until then.
Review each version at the end and draw lessons. It is recommended that questions about the timeline, budget, satisfaction, scope, negotiation capabilities, team, skills, and training be raised. Conduct the lesson-learning process two months after going live.
This stage (of evaluation) includes business activities such as preparing information for review, preparing for meeting content, conducting the meeting, and implementing lessons learned.
Tips:
Prepare for every business intelligence activity, assuming several versions will be released and not everything will be released at once.
Don't give up on event reviews and lessons learned after each activity launch.
Commentaires