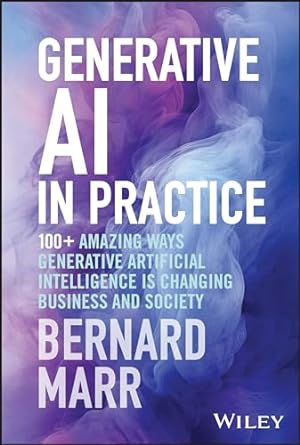
Generative AI in Practice: 100+ Amazing Ways Generative Artificial Intelligence is Changing Business and Society was written in 2023 by Bernard Marr, an expert, and author of books on artificial intelligence and innovative technological and business trends (see also related books whose summaries we have published here on Future Skills, Artificial Intelligence in Practice, and Business Trends In Practice).
Indeed, we've all probably already experienced Generative AI at some level, whether for personal or business needs, and some of us even understand the deep capabilities inherent in these tools—and yet, readers of the book will be left gaping. Marr also lays out a recommendation on how to implement it. In between, he provides practical ways of application in 12 different sectors, Including real examples.
The bottom line is that Generative AI is a revolution.
The book begins by explaining GenAI and its potential, challenges, and risks and then moves on to the main topic—GenAI applications in various sectors. It includes guidance for tomorrow morning on how to start preparing for implementation in organizations.
Marr risks writing a book with a seemingly limited shelf life since the field is evolving daily. And yet, it is worth reading now. Marr defines generative AI as one of the most powerful technologies ever put into human hands. Anyone can learn and advance their occupation today.
Understand Generative AI
To understand generative AI, you need to define AI first. Artificial intelligence refers to computational algorithms that can effectively mimic human thought processes, such as learning, decision-making, and problem-solving.
Generative AI, or Gen AI for short, is a sub-technology of artificial intelligence capable of generating new content based on patterns and structures learned from existing content.
The meaning: Generative AI is about simulating human creativity.
The content can be varied: text, image, video, audio, data, software code, games, and more.
Unlike general AI, which focuses on recognizing patterns, GenAI focuses on creating them. It is based on specific neuron networks called generative models.
Key milestones that enabled the development of GenAI:
1950s: The initial development of artificial intelligence
Early 21st Century: Big Data; deep learning; neural networks; Integrated models
20s of the 21st century: cloud computing; edge computing (distributed); IoT; Quantum computers.
GenAI creates the potential to influence our lives at the level of the individual as a person (for example, in translating information or deciding to cook a meal); at the level of professional work (for example, in summing up meetings or writing code), at the level of business models (in choosing optimal models for the situation), the services and products offered (for example, advisory mirrors); and at the level of global society as a whole (e.g. in the conservation of extinct languages).
GenAI will impact jobs. New roles will be created (e.g., prompt writers and intelligence instructors), and there are professions in which the number of employees will be reduced or eliminated (e.g., customer service representatives). However, the essence of the professions will change, and the human-machine balance in how the functions are performed will change (e.g., accountants and software developers).
Challenges and risks
There is no doubt that not all is rosy, and there are risks and challenges that must be considered and dealt with.
Ethical problems
The ethical risks are multiple. Main points
Misinformation
False information
Improper use (misuse)
Identity forgery (deepfake)
Is this supposed to prevent us from moving forward? Not. You have to know and cope and also know that:
A significant part of what is published significantly radicalizes the description of the situation.
We are already beginning to develop technological solutions for some of the problems.
Loss of human skills
Loss of human skills is a double risk:
The difficulty of specialization and the creation of skills. Since GenAI will serve as a virtual assistant for many tasks, the machine will perform tasks previously performed by apprentices and interns.
Preserving existing skills is difficult since intelligence performs them for us (as with calculators or navigation software).
As a society, it is necessary to formulate solutions for these needs and, in some cases, to accept the loss or weakening of skills.
Bias and explanatory ability
The problem of bias is not characteristic of machines. However, human beings, since the machine intensifies human biases in the content on which it is based, it becomes more significant and can pose a risk.
When GenAI is based on all the open information on the Internet, it balances itself in most cases, so the risk is small compared to the seller in domain databases.
The challenge of explanation stems from the behavior of intelligence as a "black box."
Marr refers to technological solutions that are evolving in this context. It is also recommended that the machine be asked for explanations and justifications for recommendations, as well as to ask the intelligence to exercise critical thinking about the proposal and, if necessary, to cross-reference the products with other sources of information.
Legal issues
Key issues include copyright, the legal protection of information (such as GDPR), and the need for new legislation.
There is a double question about copyright issues: a) Is it possible to rely on the information without infringing copyright? and b) whose copyright of information created with the GenAI.
There are different approaches, from a blanket ban on the use of various companies to copyright denial because of the machine's integration and synthesis based on big data, and multiple solutions in between, such as Open AI's new trend to allow artists to decide that their work will not be included as input to models.
Legislative efforts are developing, whether in the US, China, or Europe, especially in 2024; the legislation is still in progress.
Environmental impacts
Like many artificial intelligence technologies, GenAI consumes enormous computing resources and adversely affects climate and environmental problems.
The hope is that in the long run, a solution will be found, and until then, we will also be wise enough to use the response to climate problems positively, thanks to GenAI, while balancing, at least partially, the heavy price.
Implementation of GenAI across sectors
Marr offers extensive detail on a representative list of sectors to which GenAI's potential contribution could be applied, including many examples of companies and products already being implemented in practice.
Here are representative (partial!!) examples of each of these sectors:
Media and entertainment
Typical applications:
Journalism: writing articles, analyzing audience reactions, making videos.
Sports broadcasts: demonstration of moves from different angles, personalization of footage. Books and films: idea making, fiction writing, translation.
Music: creating background vocals, accompanying instruments, creating voice.
Art: Simulating works of art based on desired styles.
Organizations- examples:
Sport: A summary of sporting events.
Formula One: Simulations that measure billions of parameters for each race, insights based on photography, and more.
Software products- examples:
MidJourney
Elf Tech (creating personal music with the relevant singer's voice)
Advertising & Marketing
Typical applications:
Personalized content
Ideation, creating advertisements, marketing content formulations, preparing images, and accompanying music.
Analysis of sentiments in social networks.
Organizations- examples:
Heinz: A series of funny photos on social media demonstrating ketchup in space.
FOX TV: Create GenAI-based videos.
Software products- examples:
Adobe Firefly GenAI package
Salesforce Einstein GPT
Customer Service
Typical applications:
Managing the dialogue with the customer in search engines.
Analyze data and surface insights on the database.
Support service representatives during the call. personalized user experience, personalized recommendations, and interventions.
Create step-by-step customer troubleshooting guidelines.
Predict problems and make preliminary recommendations on component replacement or maintenance.
Quite a few customer service tasks can be fully transferred to the machine, and many others should be disassembled and done in combination with the machine.
Organizations- examples:
Octopus Energy: A bot is reported to be doing the work of 250 employees (44% of the work).
JetBlue: Assisting agents and reducing an average of 280 seconds per call, resulting in savings of 73,000 hours per quarter.
Software products- examples:
Zendesk: Assisting Agents
Twilio: Customer AI
Sprinklr Note-taking Automation
Commerce & Retail
Typical applications:
Improving the user experience on the site.
Virtual stores in the metaverse: sales aids, displaying clothes on a customer if and color match.
Advisory mirrors.
Plan an all-inclusive shopping spree.
Personal clothing design (not by an expert).
GenAI-based loyalty, promotional, and discount programs.
Automatically prepare product descriptions for your catalog.
Negotiations with suppliers.
Organizations- examples:
Carrefour: Introducing Hopla, an advisory robot for stores.
eBay: ShopBot, a robotic shopping assistant that integrates with Facebook Messenger.
Walmart: A shopping assistant that enhances the shopping experience.
Learning and education
Typical applications:
Personalized learning.
Assistance in preparing textbooks, study plans, exercises, quizzes, and tests.
Assisting teachers with feedback on students' weaknesses.
Automatic creation of follow-up questions and in-depth questions where students have difficulties.
Answering questions and difficulties for students in real-time while learning.
Recommendations for teaching staff on how to deal with behavioral challenges.
Preparation of summaries for the learner.
Assisting in learning and developing soft skills.
Organizations- examples:
Khan Academy: Personalized learning that changes questions according to learner understanding; Also helps manage the entire classroom.
Udacity: Accompanying personal learning, preparing summaries, and translating learning materials into desired language.
Software products- examples:
Quizlet GenAI: Personalized learning tutorial
Health
Typical applications:
Personalized consultation for patients.
Identification of diseases; early detection of diseases.
Identification of problems on X-rays, MRI, and CT images.
Personalized drug therapy; Preventive care.
Medical research and development of new drugs.
Improving and streamlining operational processes.
Summary of doctor's appointments.
Organizations- examples:
Medical schools of Harvard and Oxford: research purposes, examining the evolution of viruses and mutations.
Software products- examples:
Ada: Medical recommendations in different languages, depending on the symptoms (treated 13 million patients!!)
NextGen: Clinic Management
Video Games
Typical applications:
Game design: characters, setting, stories, and more.
Game software development involves developing the environment in which the games are run, automatic modeling, and the creation and synthesis of sounds and sound effects.
Create quizzes. Automate the testing phase of computer games. Provide tools and optimizations for users’ self-development of games.
Organizations- examples:
Ninja Theory
Ubisoft
Software products- examples:
Animate Anything: Animation of 3D characters.
NVIDIA: Cloud environment for gaming.
Legal
Typical applications:
Write documents’ drafts.
Legal research Disclosure and review of information.
Contract analysis (summaries, keywords, potential issues)
Evaluation of the results of a legal inquiry Legal chatbots advise.
Organizations- examples:
Thomson Reuters: answers lawyers' questions.
Macfarlanes.
Software products- examples:
Lexis+: Legal Research
Legal Robot: Simplifying documents and legal language for ordinary people.
Design & Development
Typical applications:
Speeding up drug development processes: recommendations on diseases or conditions for development around them; proposing chemicals or proteins with therapeutic potential for a specific need.
Examining the suitability of a chemical and its ability to respond well to the need.
Personalized medications for a particular person according to his characteristics. product design; 3D integrated design. identification and prevention of machine failures.
Predicting vehicle safety risks.
Assistance for academic research.
Create a Digital Twin replica for a factory or computer group.
Organizations- examples:
Merk: Assistance in drug development.
Tiffany & Company: Jewelry prototype design.
Software products- examples:
Dell: A platform for cancer diagnosis and predictive research.
Google DeepMind: Genetic mutation database and ability to investigate.
Consensus: A yes or no answer, whether a particular issue is a consensus of the academic community.
Finance
Typical applications:
Customer service (see above).
Personalized banking.
Automating banking processes: loan and credit decisions, friendly explanations for refusal, fraud detection and prediction, financial modeling, payment systems, stock exchange trading strategies, and asset management.
Compliance.
Accounting processes automation: reports, forecasting, data analysis, risk management, recommendation, and simulations.
Organizations- examples:
Bank of China
AntGroup
HSBC
Morgan Stanley
Moody’s.
Software products- examples:
Intuit: Assisting in personal tax management.
Software Development
Typical applications:
Requirements gathering.
Code writing.
Autocomplete
Perform software tests.
Bugs’ fixing.
Organizations- examples:
Freshworks: Automated code writing.
Augment code auto-completion.
Software products- examples:
GitHub Copilot.
Codacy: code-writing automation.
Data and business intelligence
Typical applications:
For over 20 years, organizations have been engaged in business intelligence and much more in data analysis, but with partial success, which is one of the main reasons why data-driven decision-making is low in scope.
Creative intelligence assists.
Data Pattern recognition
Data summary
Forecasting based on data Exploring data-driven ideas and scenarios
Data cleansing
Data visualization.
Organizations- examples:
TELUS
JetBlue
Software products- examples:
MS Power BI
MicroStrategy AI
Applying Gen AI
Applying is not trivial. It begins by cultivating an appropriate mindset and culture:
A mindset that refers to the desire for a combined human-machine process, a process for collaboration between people, adaptations and flexibility, curiosity and learning, and critical thinking.
A culture that begins with leadership, a supportive (flat) organizational structure, and an understanding that people's careers are changing and developing.
It is necessary to develop skills for decentralizing authority (for the machine), developing new skills for employees according to their specific occupation, relying on partnerships, and continuing to fight for the talents and good people who will remain in the organization.
Marr recommends appointing a dedicated board member to lead AI (rather than placing the responsibility on the CTO or VP of Digital).
Data is also a critical factor in the success of the process: treating data as an organizational resource and asset, taking care of new and up-to-date data, safeguarding organizational data, and constantly reviewing external data and information that is important to consider.
Of course, technological preparedness is required, including communication networks, data architecture, information security infrastructure, and GenAI tools suitable for the organization's needs.
It is recommended that a GenAI strategy be developed to derive the moves.
Adopting GenAI will be iterative and require learning, experimentation, falls, lessons learned, etc.
Such preparation is a crucial condition for the success of the implementation.
Is this the end of the road? Absolutely not. GenAI is constantly evolving, and many more surprises are expected whether combined with intelligence agents who will work together to obtain comprehensive intelligence (and not just independent tasks), whether for integration with robots (initial solutions are already being observed) or in direct interfaces to the brain not through a keyboard.
In conclusion, GenAI is an awe-inspiring solution, but at the end of the day, it is only a tool. When to use it, what to use it, and how to realize its potential most positively and benefit us and society—it all depends on us.
Comments