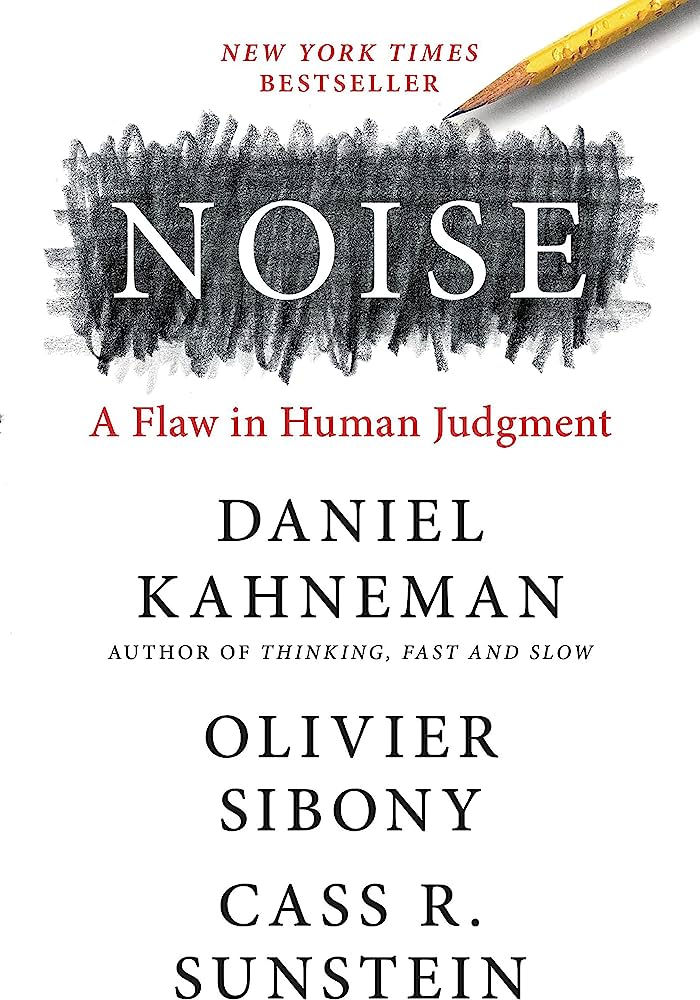
The book "Noise: A Flaw in Human Judgment," authored in 2021 by Nobel Prize Winner for Economics Daniel Kahneman in collaboration with Olivia Siboni and Cass Sunstein, delves into a phenomenon of greater significance than commonly perceived: errors in human judgment caused by "noise." Noise pertains to unexpected disparities in decisions made by individuals in professional contexts, including judges, doctors, appraisers, managers, recruiters, and social workers involved in recommending child custody arrangements during separations.
Generally, we assume that our judgment errors fall within the scope of "normal variation." If a noticeable deviation occurs, we will investigate it to comprehend its underlying causes. However, the pivotal point is that our perception of the realistic norm often holds substantial importance. This becomes apparent only when meticulously examining a vast dataset of judgments from a statistical perspective.
The book covers the following topics:
Basis: What constitutes noise in human judgment?
Reasons: What are the origins of noise, and how does it manifest?
Snapshot: How can an organization appraise the presence of noise?
Enhancement: Tools for refining human judgment.
This summary encapsulates key facets and inevitably cannot encompass the entire breadth and depth of the original work. Notably, this summary doesn't highlight nuances like the distinction between predictive and evaluative judgments, individual decision-making, clinical assessments, and more. The selected topics aim to simplify the presentation of the book's core concepts.
Like Kahneman's prior works, this book imparts profoundly valuable insights that should motivate organizations to assess and enhance their operational methodologies, starting today.
Basis: What constitutes noise in human judgment?
When decisions are made, two scenarios are possible:
The decision is correct.
The judgment contains errors.
When expressing opinions, it is acceptable and beneficial to encounter a diversity of viewpoints that reflect people's values, attitudes, perceptions, and preferences. However, this book needs to focus on those aspects. It centers on professional judgments—such as a doctor's diagnosis or a judge's sentencing—where we anticipate a combination of accuracy (correct decisions) and a degree of uniformity or at least reasonable consistency in the outcomes.
Errors in professional judgments can be broadly categorized into two primary types:
Errors originate from cognitive biases embedded in our minds (e.g., the anchoring effect—where initial information heavily influences us). These biases lead to similar products, yet detecting them can prove elusive. Identifying these errors becomes challenging when biases cause everyone to veer in the same direction.
Errors arise from systemic noise*, encompassing various decisions made by professionals who we expect to exhibit similar, well-informed judgments. However, reality doesn't always align with this expectation. Unlike biases, noise is more detectable, even if uncertainty surrounds the correct decision. It surfaces as substantial variability in cases where we expect limited diversity.
* Noise represents the unwanted variance in individuals' judgments unrelated to biases.
It is essential to clarify that the book does not delve into noise within judicial decisions. Understandably, the scale of this phenomenon can be extensive (context-dependent). Notably, these errors don't offset one another; incorrect medical treatment and erroneous premium evaluations are harmful, highlighting the critical impact on individuals.
The explored types of noise include:
Noise level pertains to situations where two individuals evaluate the same cases. Still, varying perceptions of the rating scale lead one to be consistently more lenient while the other remains strict. Instances also arise where two people interpret the rating scale differently (e.g., defining "outstanding" or establishing the threshold for extenuating circumstances). Rank noise relates to fluctuations in the average rank of judgments made by different evaluators.
Stable pattern noise denotes noise that an evaluator consistently applies, such as a constant positive bias towards cases reminiscent of close family members. Pattern noise encompasses the variability in judgments across different evaluators for specific topics.
Occasional noise arises from external factors like weather, personal stress, exposure to news, time-of-day fatigue, or preceding case influences. In contrast to pattern noise, random noise leads the same individual to provide different judgments when facing the same instances again (without recalling the initial judgment).
Considering the relative extent of these noise sources, it's reasonable to assume that stable pattern noise predominates.
Reasons: What are the origins of noise, and how does it manifest?
Noise within professional judgment decisions can arise from a multitude of factors, including:
Variances in selective attention and recall among individuals.
Absence of formal processes coordinating decision stages and components.
The disparate weighting of decision-influencing elements by different individuals.
Varied evaluations of the same part (e.g., assessing someone's qualities as a team member).
Differing skill levels among professionals.
Influence of initial impressions regarding the person or case under scrutiny.
A sequence of case examinations.
Social influence results in consensus or even group polarization and extremism.
Fluctuations in brain activity among different individuals.
Reluctance to employ automated tools and established rules, notwithstanding their imperfections (accompanied by a degree of skepticism, considering their error rates consistently prove lower than human errors).
External factors such as fatigue, stress, time of day, and mood impact decisions.
Objective ignorance stems from an intuitive sense characterized by internal satisfaction upon reaching a solution and concluding the judgment process, as the book's authors describe.
Experts' overconfidence.
Denial of ignorance: the notion that subjects entirely resistant to reasonable intellectual prediction (due to inherent uncertainty) can still be evaluated. Often, individuals who comprehend a subject assume that their understanding of a causal chain of factors enabling specific behavior equips them to predict outcomes. However, retrospective explanations need to be revised to allow for accurate future predictions.
Certain individuals' heightened sensitivity to specific aspects of a case.
Errors in judgment can also arise due to various biases, including pre-judgment, conversion of complex issues into simpler parallels, excessive coherence, order of information reception, our limited capacity to encompass scales beyond a specific range (typically exceeding 7), difficulties in interpreting intricate rating scales, group influence, and more.
Snapshot: How can an organization appraise the presence of noise?
Determining the accuracy of a decision in hindsight is feasible in some instances, although only sometimes applicable. Regardless of the context, the extent of noise can be evaluated, whether or not the decision's correctness is known, through consistent testing procedures that organizations can undertake. In such evaluations, organizations scrutinize disparities in decision outcomes. Alternatively, when products are known, the organization calculates the average sum of squared errors (this metric accords equal importance to mistakes in both directions while assigning greater significance to errors significantly deviating from the desired outcome).
By examining the peaks in this manner and adeptly discerning biases, the residual errors are ascribed to noise. Organizations must address all forms of errors—both tendencies and noises—utilizing a diverse array of methods, as elaborated upon in the forthcoming chapter, to enhance human judgment in both dimensions.
The principal testing methodologies encompass the following:
Computer-based assessments are reliant on extensive datasets. These aid in identifying diverse noises, particularly intermittent noises that might be challenging to detect through alternative means.
Noise audits involving human experimentation.
Implementation details for noise control:
Formation of a professional and managerial team tasked with executing the audit.
Creation and refinement of cases and accompanying content for audit utilization.
Collaborative definition, alongside managers, of expected levels of acceptable non-conformity and cost assessment of errors; Formal documentation of these expectations.
Experimenting within the designated units, categorized under decision-making research.
A thorough analysis of outcomes and presentation of derived conclusions.
Enhancement: Tools for refining human judgment
A diverse array of tools exists to enhance human judgment. These tools encompass both computerized and human-based methodologies, with some combining both elements.
While the ensuing list of tools is presented, more is needed to advocate for a complete transition to computer models, removing human involvement from judgment processes altogether. Nonetheless, such an approach could significantly diminish the noise level and improve judgment outcomes in numerous scenarios.
It's important to note that there's no one-size-fits-all solution provided here. Rather, a toolkit is offered, encouraging organizations to explore how to integrate its components and employ them to curtail the extent of errors. These errors, unfortunately, have been demonstrated to be notably substantial according to extensive research, hence dismissing their significance would be misguided.
Key facets of computerized tools encompass:
Rule-based model: This employs straightforward rules defining the decision-making process based on predetermined predictors (some assessable by machines, while others rely on human evaluation).
Multiple regression-based models involve calculating diverse weights for predictive factors by comparing results against an objective function (the correct answer). The model is constructed based on these weights.
Simple model (linear regression model): This prediction model linearly combines the values of predictive factors.
Artificial intelligence/machine learning-based model: This pertains not only to linear patterns but also intricate pattern recognition facilitated by machine learning.
In many instances, it has been revealed that the simple model or even random selection outperforms human judgments on average in terms of noise. Consequently, for most situations, employing a simple model that yields favorable outcomes and is straightforward to implement is recommended.
The key human methodologies for enhancing decision hygiene include:
Selection of intelligent judges with active mental openness: The unequivocal conclusion is that general mental aptitude significantly contributes to the quality of performance in professional judgment scenarios. Active open-mindedness involves actively seeking information that challenges existing assumptions.
Decision observer: Designating an external individual to detect signs of errors.
Instruction: Particularly relevant in fields like:
Teaching statistical literacy and probabilistic thinking
Understanding cognitive biases
Control over information disclosure order: Preventing premature impressions and judgments stemming from information desire. For instance, refraining from divulging circumstantial evidence or suspicions to a forensic expert. Meta-information's impact influences both perception and interpretation of observations.
Aggregation of independent and diverse judgments: Incorporating diverse and independent judgments based on the concept of crowd wisdom. Several models for implementation exist, with the simplest (effectual in most cases) being averaging. Ensuring judgments are genuinely independent, acquired autonomously, and aggregable is crucial.
Group judgment: Encouraging forecast producers to engage with opposing viewpoints, subsequently revisiting decisions after such engagement (requiring mental openness).
Judgment guidelines: Offering explicit standards and/or rules for the judgment process. The authors contrast standards, which afford more leeway for human judgment, and rules. The definitive preference between the two remains contingent upon organizational discretion, accounting for factors such as issues at hand, case volume, decision significance, and error cost.
Common external-scale-based terminology: Establishing consistent terminologies and scales for judgment processes. Introducing external scales outlining the parameters for each assessment level. Forced ranking is also an option (evaluating judgments relative to adjacent case judgments).
Decomposition of judgment into component evaluations: Applied, for instance, in enhancing candidate acceptance assessments. The approach involves focusing on a few (preferably no more than four) independent components, soliciting separate evaluations for each, and subsequently deriving an overall score (either human or average). Integrating holistic judgment intuition occurs only post-individual component grading.
As highlighted, no single tool emerges as the ultimate solution for judgment improvement. The book's authors recommend experimentation, refinement, and combining different tools. The book showcases examples across diverse domains, ranging from expert judgment within organizations, forensics, medical directives, forecasting, candidate selection, and strategic corporate decisions.
The authors propose an overarching strategy termed the "mediated evaluations protocol," highlighting these key components:
Agreement on the decision-making approach.
Identification of pivotal decision-influencing factors (referred to as "mediating assessments"), which should be relatively limited and independent from each other.
Mobilization of a team to gather data on each distinct key factor.
Comprehensive decision process comprising three sub-phases:
Individual judges' evaluation of each element is based on the gathered data.
Collaborative team discussion encompasses the exchange of divergent viewpoints and arguments.
Re-voting by each judge, followed by averaging.
While tools are provided to mitigate and lessen noise, the authors refrain from advocating complete noise elimination:
Noise reduction entails resource expenditure. The benefits of noise reduction need to be weighed against associated costs.
Complete noise elimination, such as through algorithmic introduction (decision mechanization), could hinder the organization's adaptability to changing variables.
Algorithms might achieve noise-free outcomes but could inadvertently amplify bias errors.
And finally... human judgment fosters respect for the subject of judgment, enabling the application of values like compassion and equitable treatment for exceptional cases. Of course, human judgment also affords respect to the judge, who might occasionally feel overshadowed by automation, a sentiment that warrants consideration.
Remember - the objective of judgment is accuracy, not personal expression. Ingeniously integrate human judgment with human and automated noise reduction tools. Strive for an optimal equilibrium to achieve heightened success.
Comments