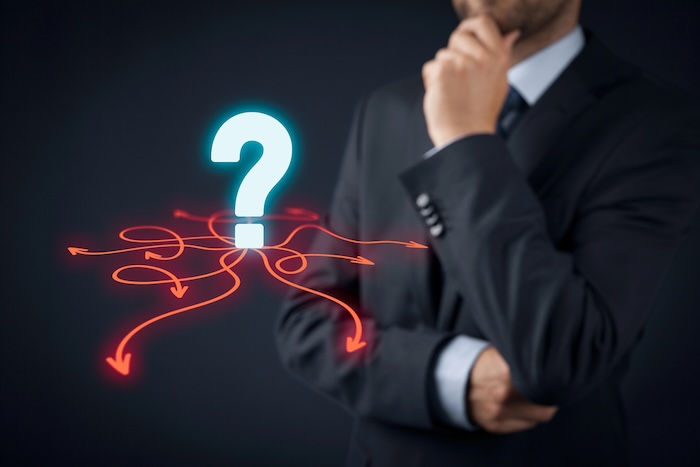
Do you know Linda?
Linda is an open and talented young woman. She graduated with a degree in philosophy. As a student, she was very concerned with discrimination and social injustice. She used to participate in protests, violence, and more. Which of the following two options is more likely?
Linda is a bank teller.
Linda is a bank teller and active in the feminist movement.
Most people choose the second option because it fits better with their image of Linda as an active student. Still, in doing so, they ignore the laws of statistics: the probability of the first option is greater than the probability of the second option since feminist bank tellers are a subset of bank tellers (out of all bank tellers, some are likely to be feminists, and some are not).
Another question: Which would we recommend more, a new drug with a 50% success rate or a new drug with a 50% chance of failure? We recommend the option of success even though the probabilities of failure are equal.
Wise and good people have already proven (and even received prestigious awards) that people are not rational.
Due to information overload, we use "mental shortcuts" in decision-making processes, which are estimates (professionally called heuristics). We thus are exposed to cognitive biases like those mentioned in the examples above.
How can we still overcome these biases? We'll use objective decision-supporting metrics.
The fear of biases and decision-making under conditions of uncertainty raises the need for "objective evidence" serving as "confirmation" for a decision or behavior, and sometimes even for one social norm or another. We attribute great importance to metrics in our lives to such an extent that the common saying is, "What isn't measured doesn't exist.”
The use of objective metrics helps solve the bias problem and reduce the feeling of uncertainty, but it also raises other challenges that need to be addressed. What are these challenges, and how are they connected to BI systems? The article will discuss all this and more.
BI Metrics and the Decision-Making Process
To understand how metrics are used, let's pause briefly to understand how we make decisions.
How we make decisions depends on circumstances, the quantity and quality of data, and our tendency to make decisions in one way or another. There are three decision-making processes:
A long and comprehensive decision-making process based on data: Business organizations (as well as individuals) prefer to make decisions this way, as decision-making is perceived as rational due to objective metrics. In a world of information overload, this process is particularly impractical.
A short and non-comprehensive decision-making process based on assessment: This process is intuitive and biased and, as mentioned earlier, less acceptable, at least in the business world.
The most common of the three is a decision-making process that combines the two—a "semi-rational" process, a compromise between the long process and the short process.
How can we still make decisions rationally? In any decision we make, there will likely be an intuitive element to some degree or another. However, we can reduce the intuitive part and increase the part based on metrics without needing a long process or feeling "drowning in a sea of knowledge" by using intelligent metrics. Innovative metrics are integrative and qualitative data (defined as relevant metrics) produced in BI systems. Innovative metrics provide comprehensive yet concise information (according to specifications) so it doesn't overload the decision-maker with information.
Examples of using innovative metrics:
Project risk assessment: Using innovative metrics to base the initial evaluation and determine whether risks materialized.
Determining business strategy: Should we develop a product, launch a campaign, or acquire a company?
Ongoing activity: Metrics reflecting customer preferences will help us offer an appropriate deal or arrange products properly in the store.
Performance evaluation: Data on usability in the organizational portal, sales data, and more.
Control metrics: ROI or VOI?
The accepted metric for evaluating investment efficiency is the ROI (return on investment) metric, calculated as the ratio between the investment (usually financial) in a specific action versus the profit derived from that action in retrospect.
Today, organizations are not satisfied with using just the traditional metric (ROI) because elements like sharing, innovation, knowledge management, changes in work processes, professional communities, and increasing productivity are beginning to be perceived as essential factors that raise the organization's competitive value. All these are reflected in a control metric called the VOI (investment value) metric.
The differences between ROI and VOI are superficial. ROI is based on tangible returns, such as reducing expenses, increasing returns, and opening new markets. The ROI metric is objective and based on traditional and concrete metrics (although the assumptions leading to ROI may be subjective and judgmental). In contrast, the VOI metric measures the overall value of "soft" and vague returns, so it is subjective and difficult to measure with the same degree of accuracy as the ROI metric.
And what's the connection to BI metrics?
The BI metrics will be used to calculate the ROI metric (for example, sales return data as a return on investment for a new campaign). Still, qualitative analysis of BI metrics (for example, analysis of usage data for knowledge-sharing solutions) will help us evaluate the VOI metric so that although it's an intuitive assessment, it will be based on solid data, raising the evaluation's validity and reliability.
The Challenges in Using Metrics
As mentioned earlier, the use of metrics helps in solving the bias problem but raises other challenges:
The chosen metrics don't necessarily reflect an accurate measure of what we intended to measure (in professional terms, it's customary to say that there's a problem with the validity and reliability of the measurement). This may be rooted in inaccuracy in characterizing the metric as a response to the measurement need (validity) and in presenting unrefined and outdated data (reliability).
Objective data has subjective value: Metrics usually express quantitative data, but their use depends on how they will be analyzed (for example, data on grades will be reflected in the system, but the decision of "what is a good grade" is a subjective decision). Data analysis will add "color,” but it's based on human thinking, which is susceptible to biases.
The need to use objective metrics may create overuse of data and dependence on technology, sometimes expressed in the removal of responsibility from the decision-maker and absolute reliance on the data (for example, hiring an employee solely based on selection tests conducted at an external assessment institute, and giving little weight to the personal impression of the decision-maker and recommendations about the candidate). Regarding BI metrics, the dependence may be more significant, as these are "smart" metrics. In this context, the level of accuracy in characterizing the metrics is critical.
In conclusion,
We can never escape, and it's also advisable not to do so from human thinking based on subjective assessment. However, we can minimize the influence of biases inherent in such an assessment by being aware of the existence of biases and by incorporating objective metrics in decision-making processes. Given information overload, innovative metrics produced from BI systems will provide the most comprehensive information we can get. Still, it's also recommended that we adopt a critical approach towards them and use them wisely as decision supporters, not as a substitute for the most innovative system created so far - the human brain. Happy measuring.
Comments